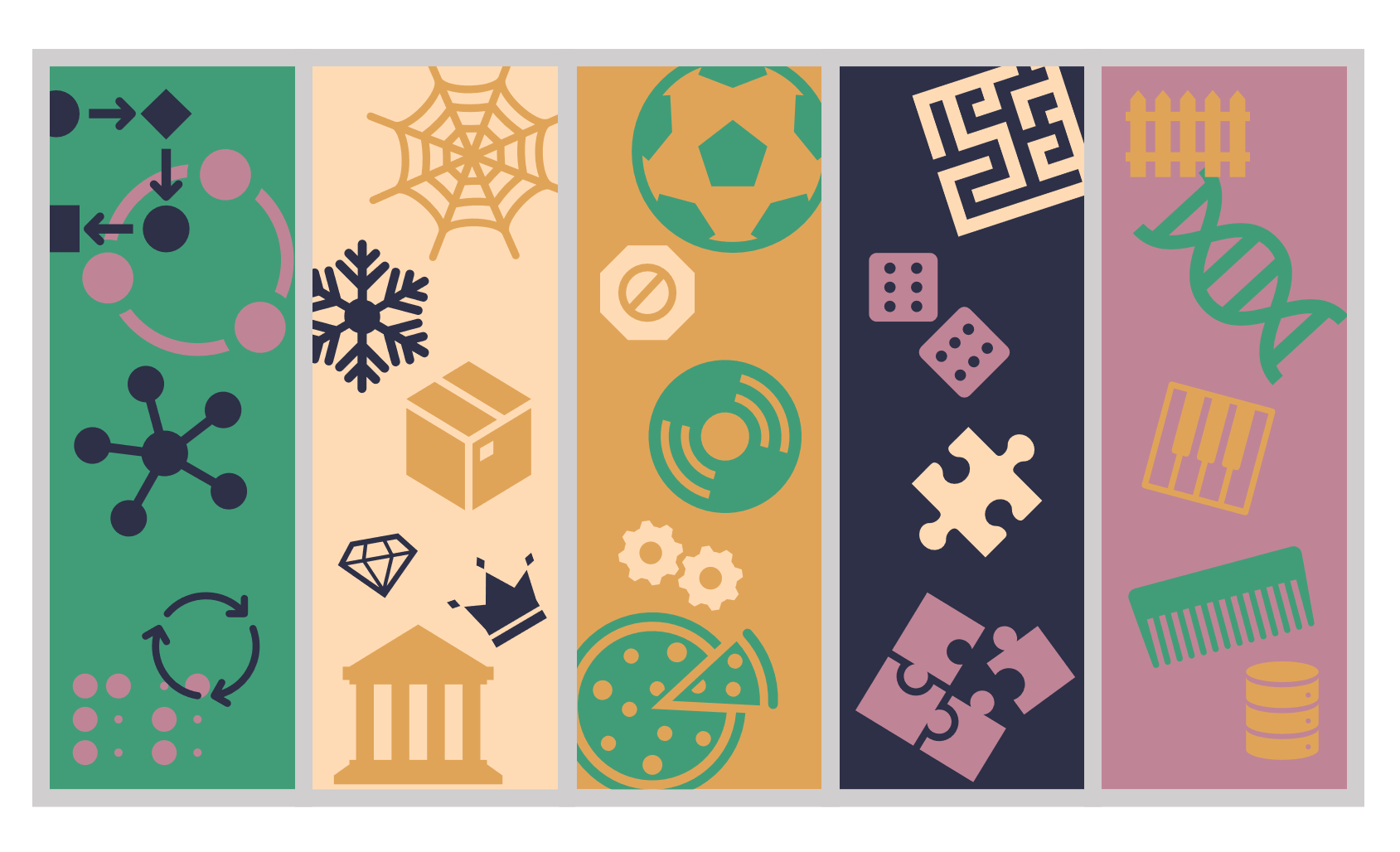
Geometric computing is crucial for many areas of application, concerning the extraction of topological and geometric information from shapes, scenes, and sequences, as geometric representations offer concise and intuitive abstractions, providing valuable methods for modeling, synthesis, compression, matching, and analysis. Recently, large foundation models have provided unprecedented opportunities for geometric computing and 3D vision, as shown in 2D to 3D content generation and open-vocabulary segmentation. We seek to bring together researchers from 3D computer vision, computational geometry, computer graphics, and deep foundation models to foster discussions on the latest breakthroughs, existing constraints, and future opportunities to develop a foundation model for geometric computing.
Our topics include but are not limited to:
- Geometric computing techniques and their benefits to deep learning
- Geometric losses and regularizations for model training and fine-tuning
- Geometric feature learning on large-scale data
- Geometry with open-vocabulary perception and interaction
- Solving discrete and continuous geometric problems with large models
- Foundation models for 3D content creation, perception, and geometric computing
- Trends, opportunities, and future topics on geometric learning with large models
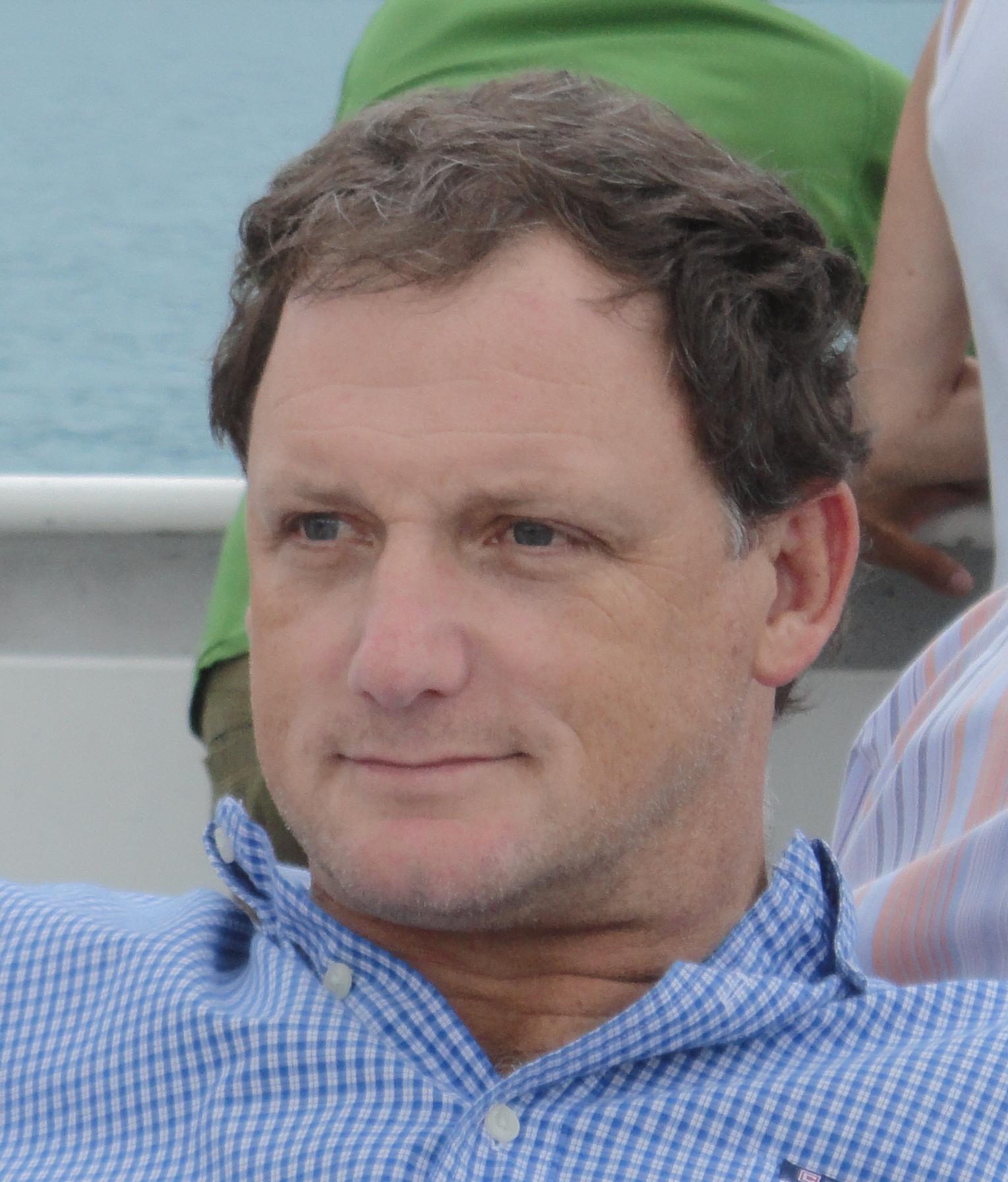
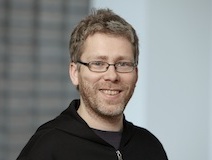
ETH & Microsoft
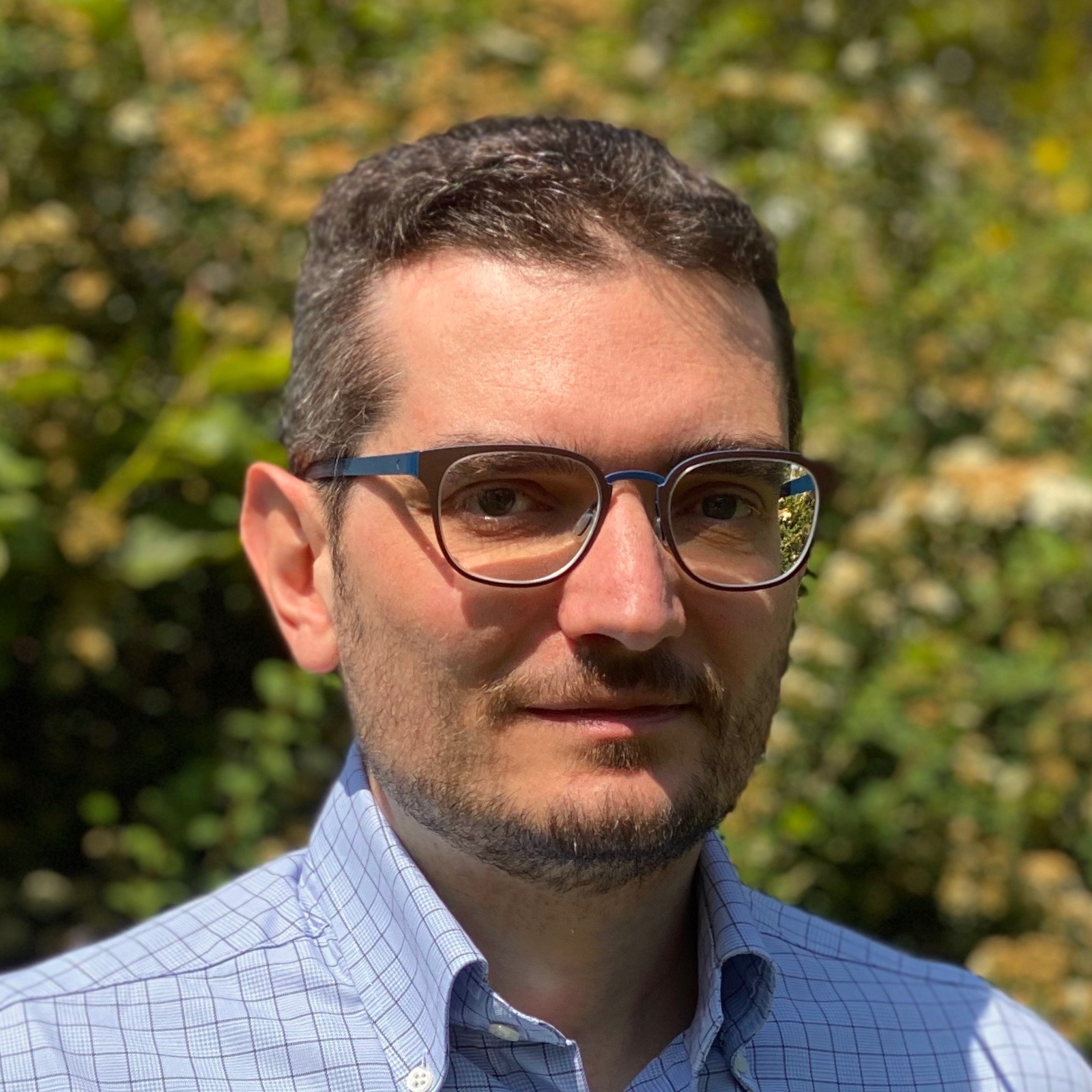
Oxford
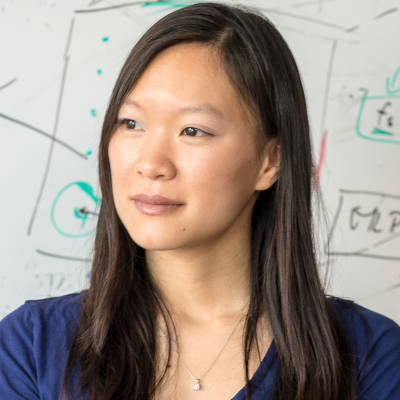
TUM
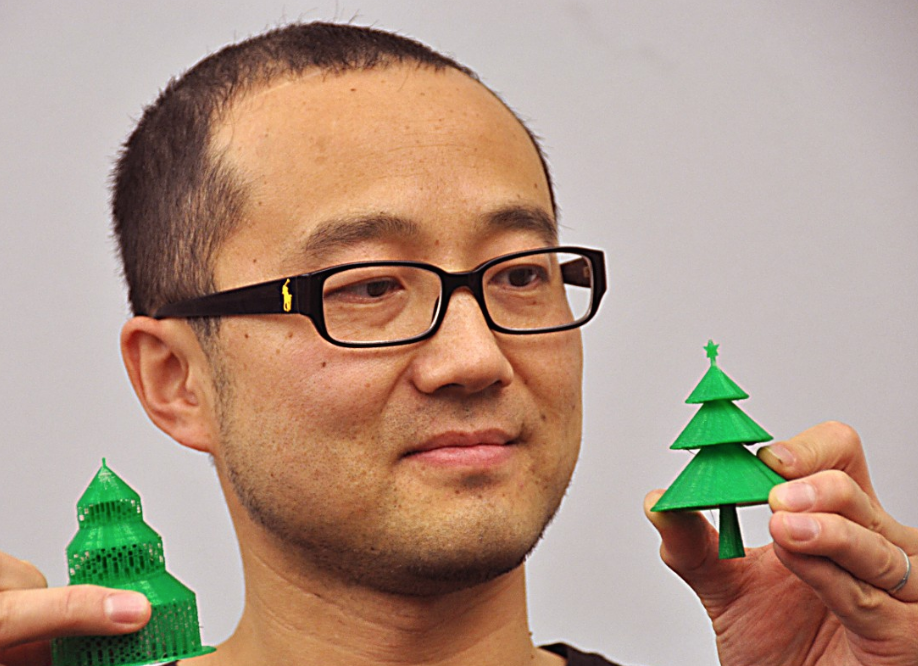
SFU
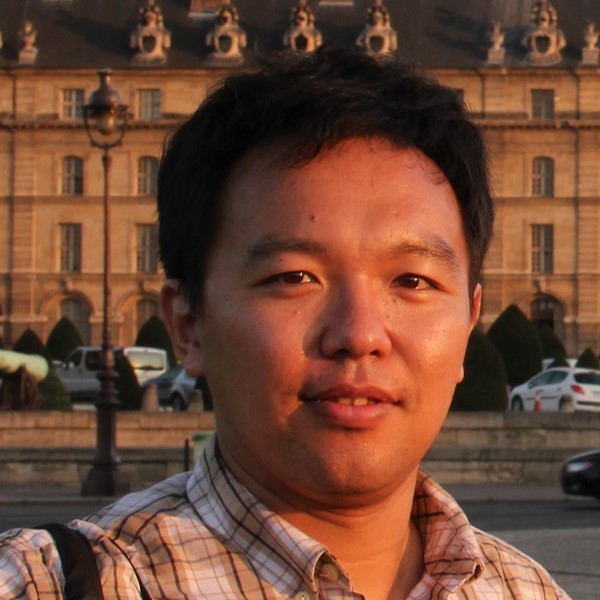
UT Austin
Welcome and Opening Remarks: Leonidas Guibas | 14:00-14:10 |
Keynote Talk: Hao (Richard) Zhang | 14:10-14:45 |
Keynote Talk: Marc Pollefeys | 14:45-15:20 |
Keynote Talk: Qixing Huang | 15:20-15:55 |
Coffee Break | 15:55-16:10 |
Keynote Talk: Andrea Vedaldi | 16:10-16:45 |
Keynote Talk: Angela Dai | 16:45-17:20 |
Keynote Talk: Tom Funkhouser | 17:20-17:50 |
Closing Remark | 17:50-18:00 |
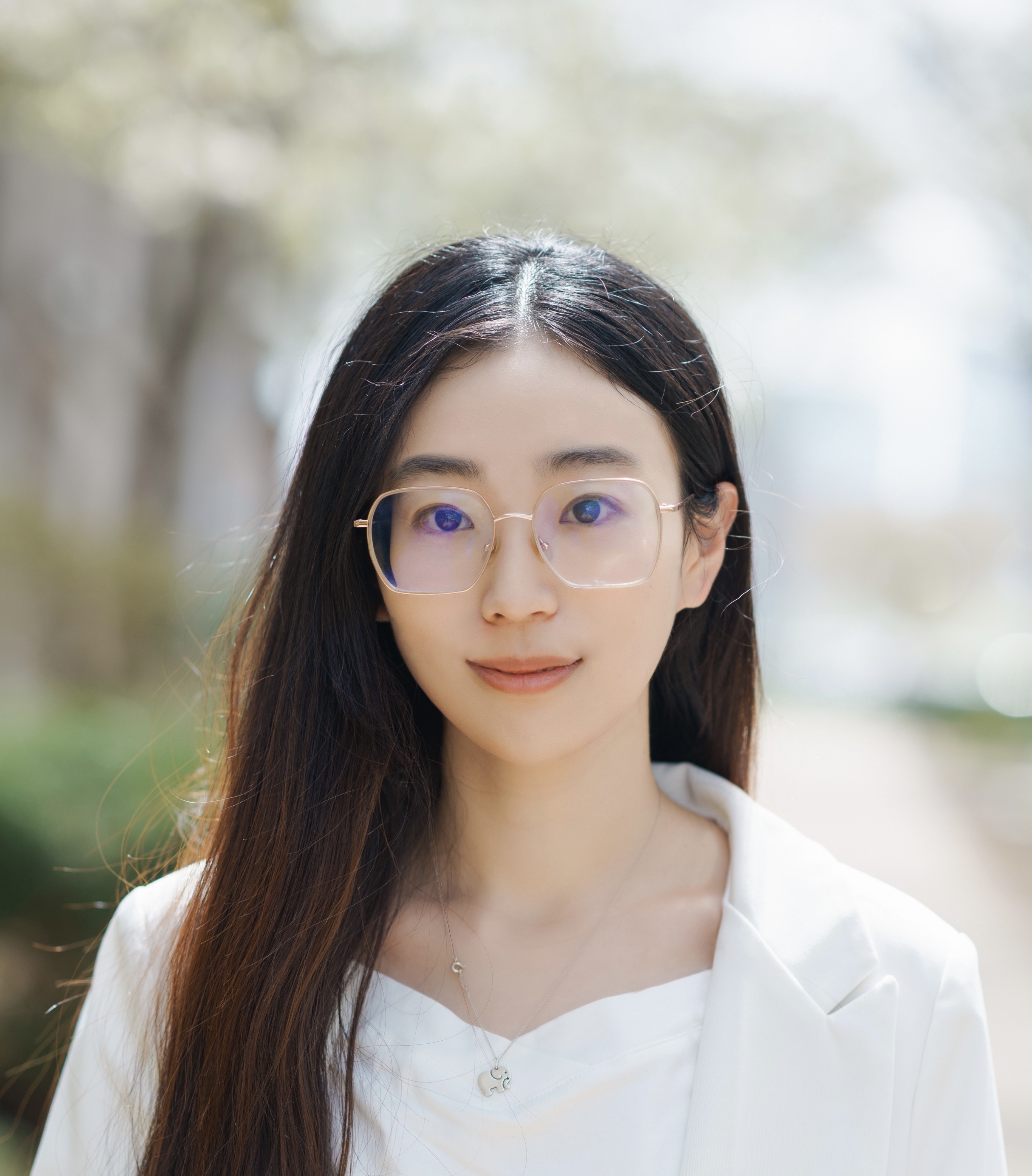
MIT
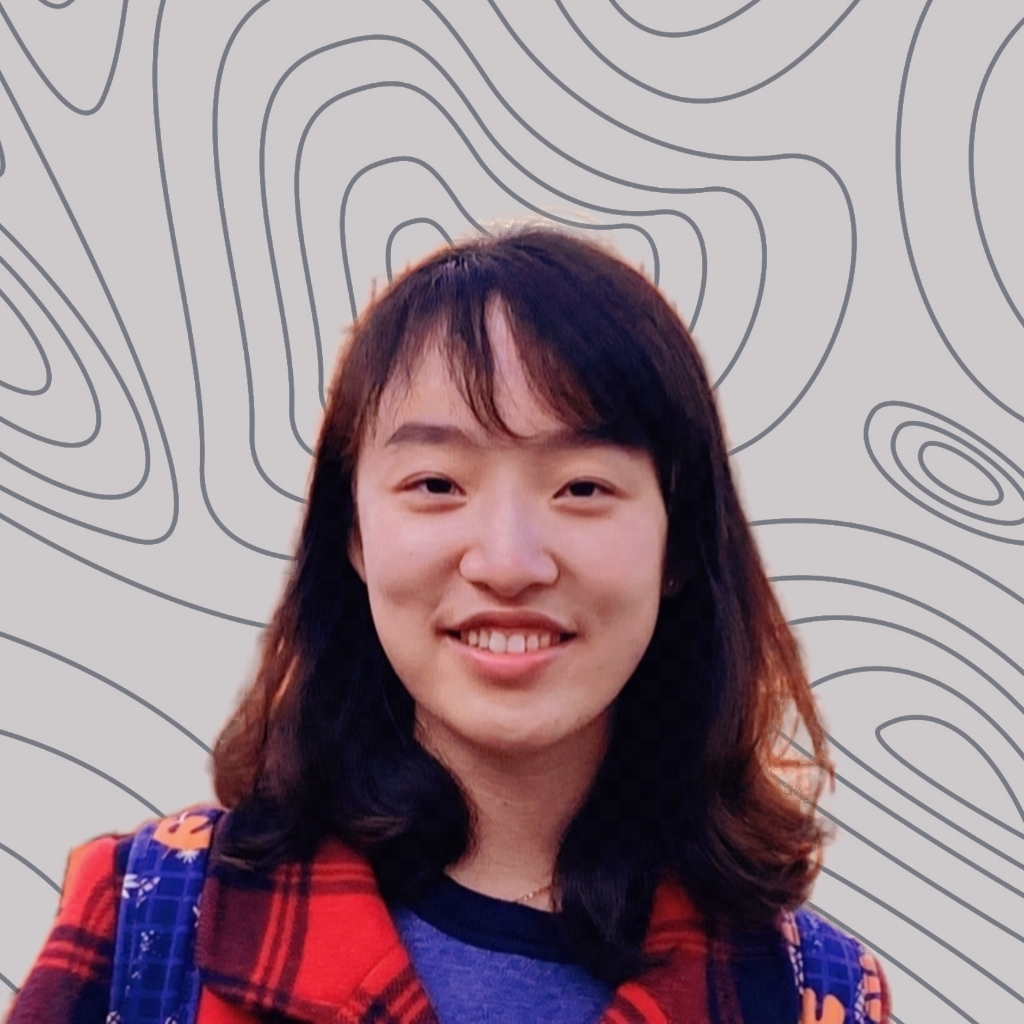
Stanford
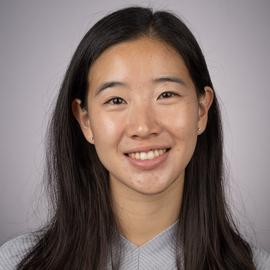
Cornell
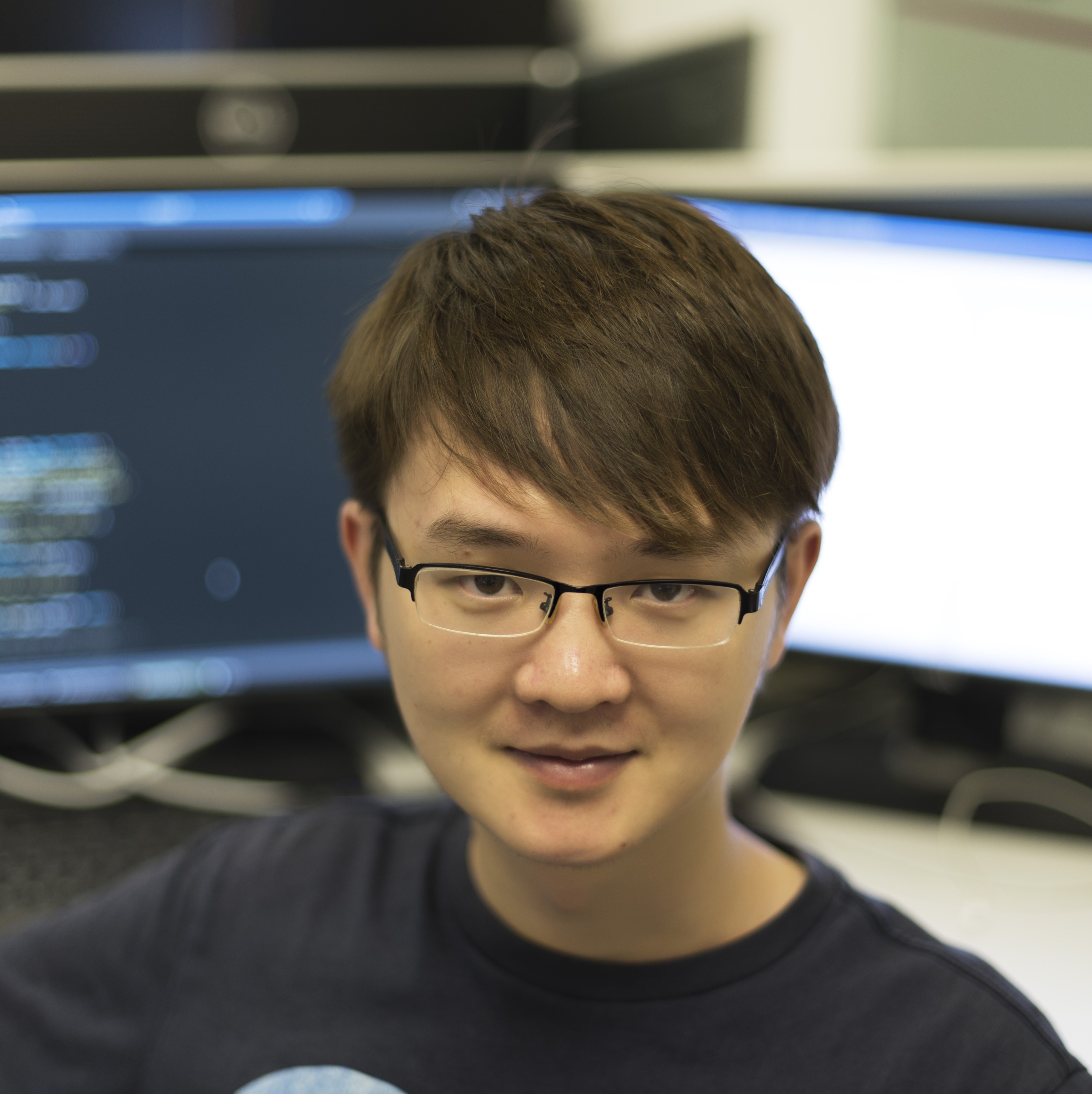
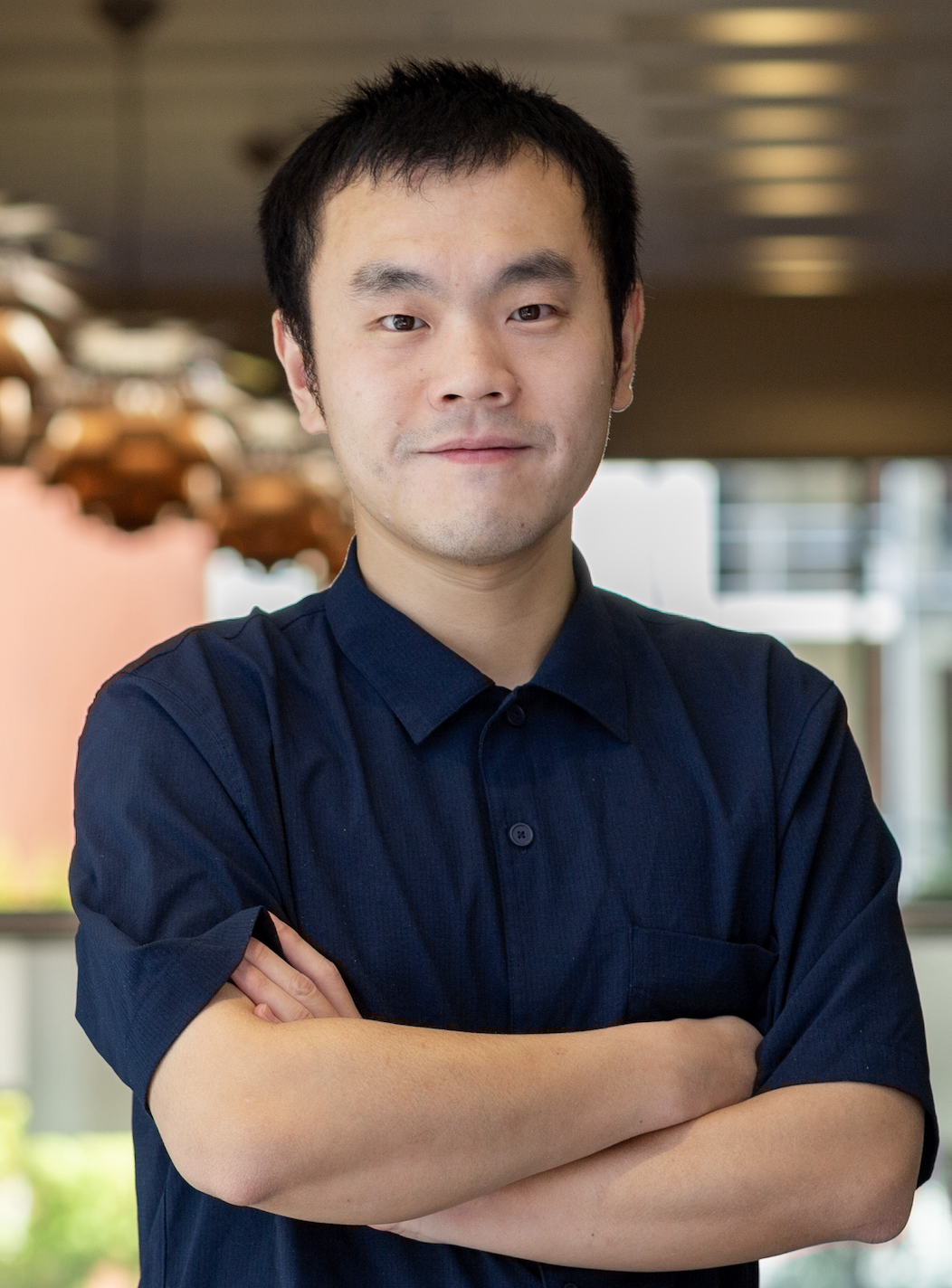
USC & NVIDIA
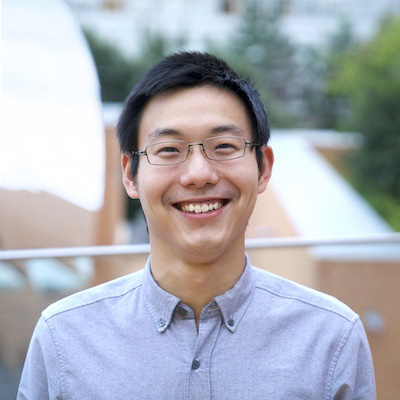
Stanford
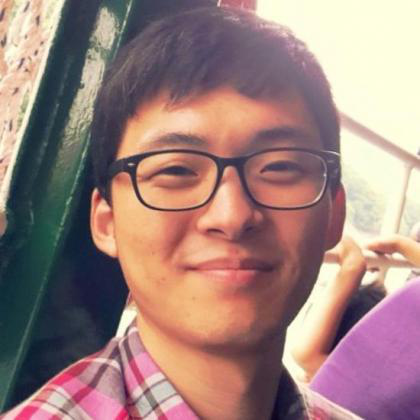
KAIST
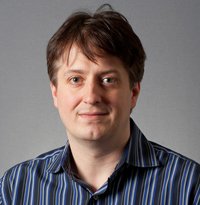
MIT
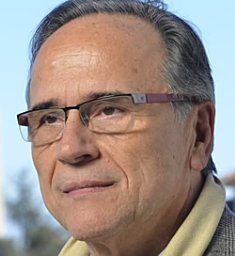
Stanford
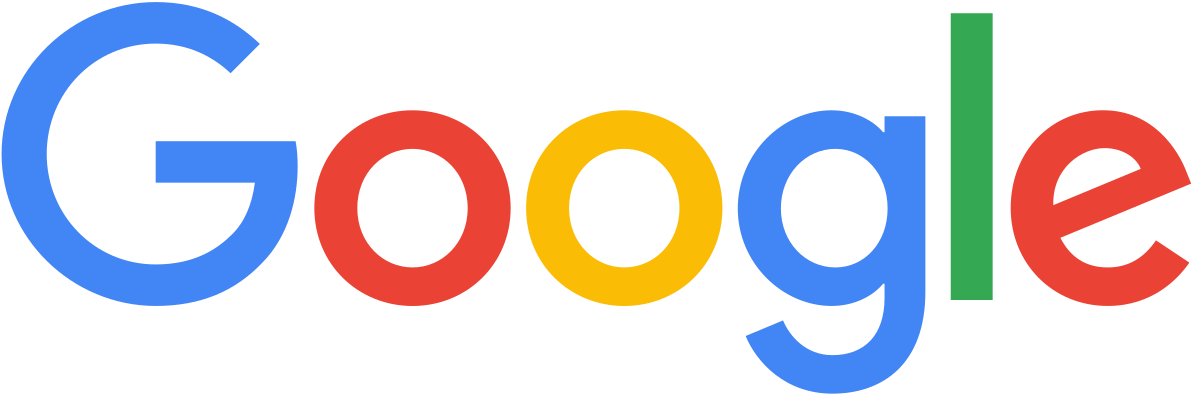